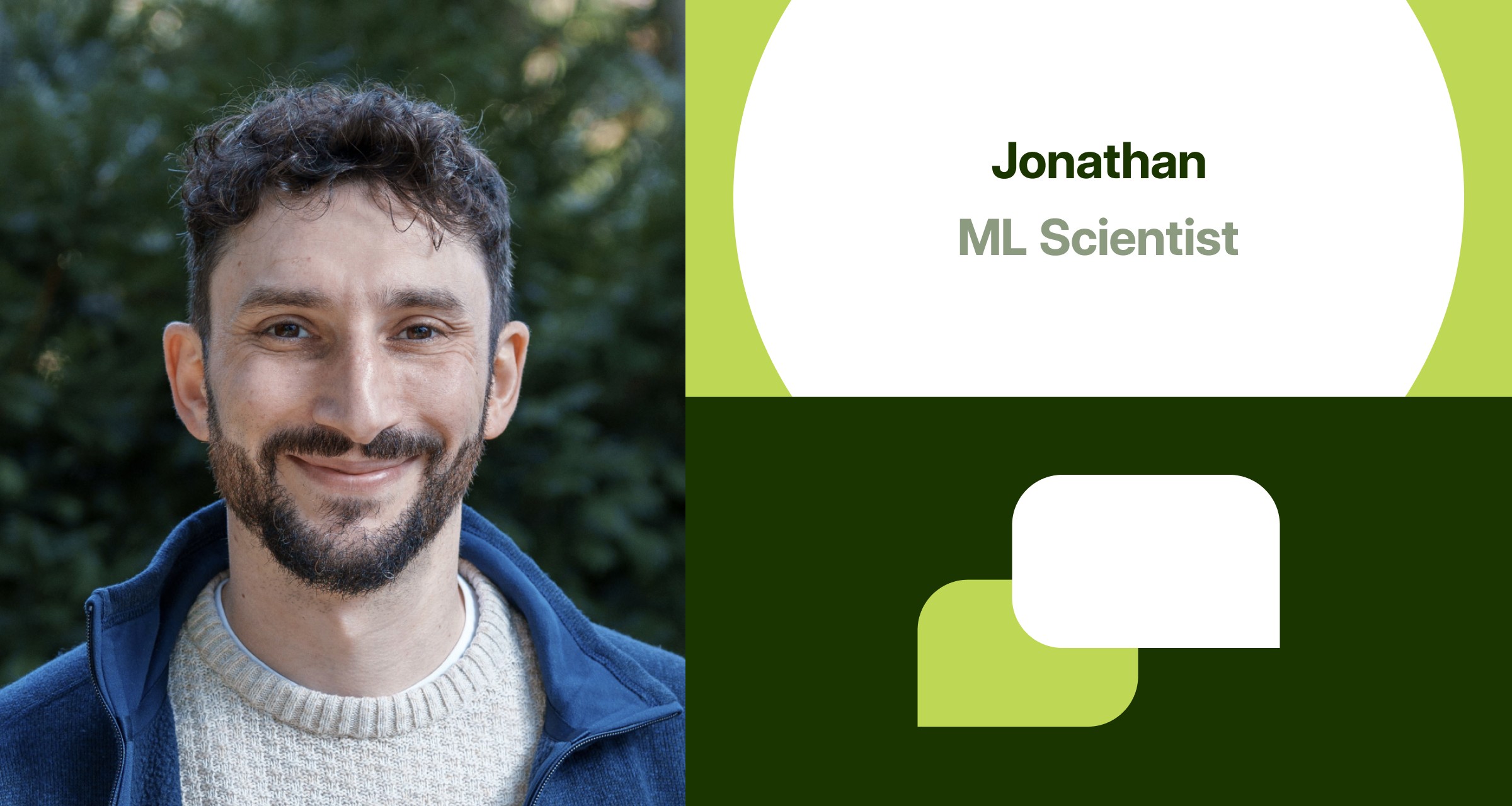
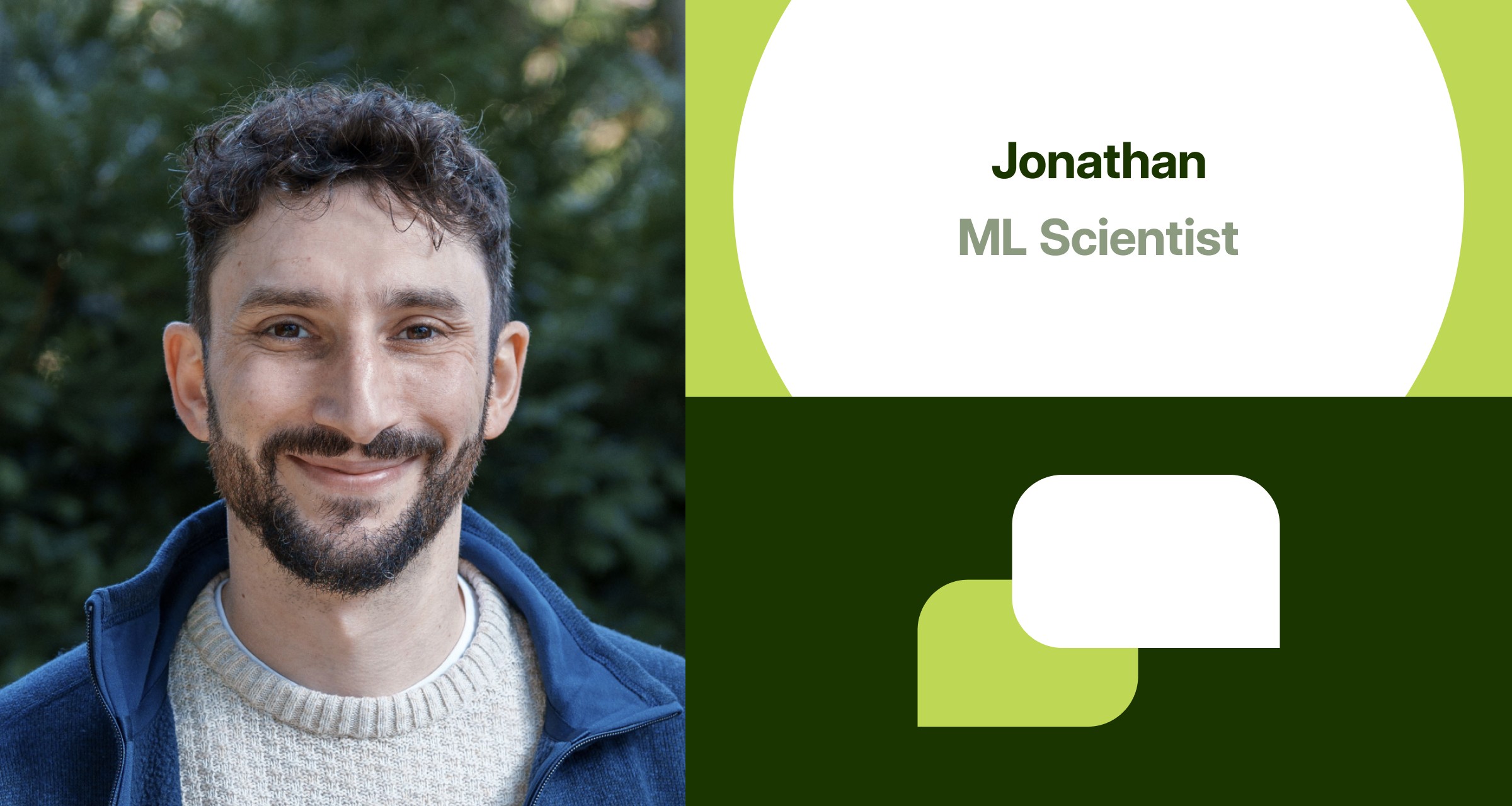
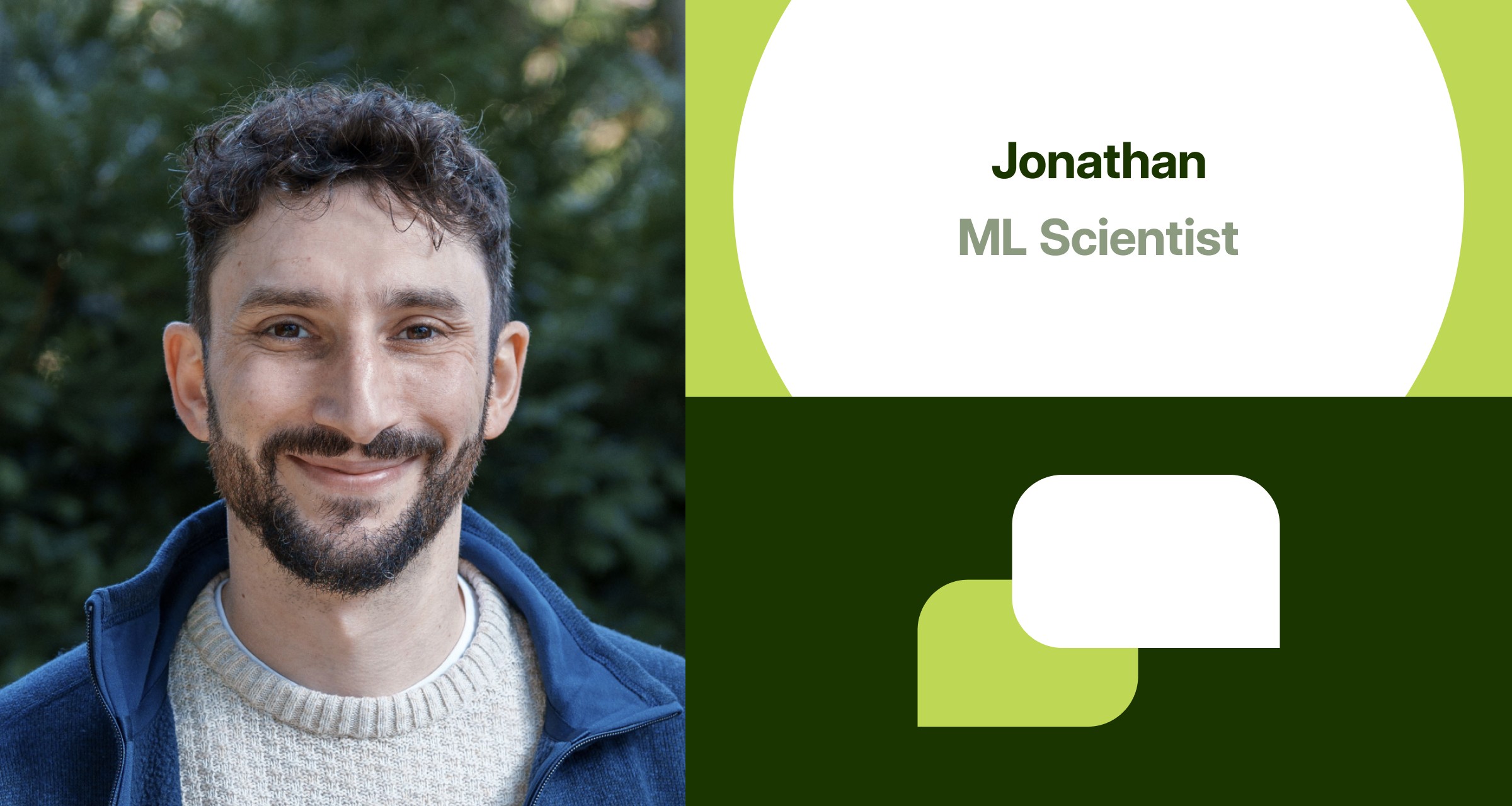
Synthetic Symphonies: How an Audio Expert is Revolutionizing Biotech with AI, an Interview with Jonathan.

Jelle Prins
May 9, 2024
May 9, 2024
Before the interview series with Cradlers kicked off, a colleague asked me an interesting question: "Do you want to interview a biologist or an ML scientist?" My response was short: 'It doesn't really matter to me,' and had two reasons behind it. Firstly, I had never conducted interviews before, so whether the conversation was about cutting-edge biology or advanced machine learning, I was bound to learn something new. Secondly, the biotech industry has entered a transformative era where the lines between life scientists and tech experts are blurring. Biologists are embracing technology and exploring computational methods, while engineers are delving into the complexities of life sciences. This merging of fields means that regardless of who I interviewed, the conversation would explore a fascinating intersection of knowledge and innovation.
Following that profound monologue in my mind, a common occurrence after discussions or altercations, where one creates the most fitting and extraordinary argument—a masterpiece worthy of a Pulitzer Prize in Explanatory Reporting—merely two weeks later, I found myself in a Google Meet session with Jonathan, Cradle's resident ML scientist. Or, as Google labeled him, "CH-ZRH-BLE-4-Rosalind Franklin," as each meeting room at Cradle is named after pioneering women in science and engineering.
Harmonizing disciplines
Jonathan is the ideal example of the convergence between technology and biology. His background is an eclectic mix of music, technology, and now biology—a physicist, music producer, ML scientist, and more.
When I asked him how he ended up with such an intriguing combination of skills, Jonathan revealed that his early years were all about music. "Music came first," he said, recalling how he began playing the violin at five years old. His parents were professional classical musicians, and his sister is now a professional singer. Despite his musical upbringing, Jonathan was drawn to STEM subjects, describing himself as "the oddball" fascinated by math, science, and technology.
Although Jonathan decided to study physics as a teenager, he quickly found himself questioning that choice once he entered university. "I found myself at university, enrolled in physics, and I was like, what the hell am I doing here?" he said with a smile. To find a new direction, he interned at SWR, a German government radio station with multiple orchestras and a studio for experimental contemporary live electronic music. "Lots of noise, screeching, weird sounds, and things like that," he described. This experience uncovered something unexpected: the resident sound directors knew more about physics than he did. "I was a physicist, and they were musicians, but they knew more about physics than I did," Jonathan laughed.
This realization led him to also study music informatics at a conservatory, blending technology and music. After graduation, he pursued music production, but the routine eventually wore him down. "It's the same thing every weekend: you go somewhere, you sit backstage, you wait for the sound check, you do the sound check, you play the concert, you go to the hotel, you go to the next venue, and so on," he said, describing the monotonous cycle that made him rethink his path. The repetitive nature of the music industry pushed him back to academia, where he earned a PhD in computer science.
Pioneering ML for audio
During his PhD, Jonathan initially focused on multi-microphone setups for large venues and events. At some point, he realized that machine learning could solve many of the problems he was tackling. "The tasks I was painstakingly attempting to figure out were far more effectively solved by machine learning," he shared. This realization prompted Jonathan to shift focus, allowing him to secure additional funding, including a grant to form a small team and become a pioneer of ML research at his university.
"We established the Institute for Applied Artificial Intelligence during that period, and it was fantastic," he explained. "It was mostly graduate students and professors, and we didn't do any teaching—just pure research. I was essentially the audio guy. We had people working on computer vision, natural language processing, and analyzing Japanese comics from an ML perspective—quite a range of disciplines, including some in medicine. It was an incredibly interesting time."
From audio to pharma
After completing his research at the institute, Jonathan realized his ML skills could have a broader societal impact beyond audio applications. He considered focusing on either life sciences or environmental sciences, but personal and family reasons steered him toward medicine. "Unfortunately, I've been in very close contact with a lot of medicine, so it really got me hooked," he said, adding with a grin, "The research, not the meds."
It was a great time to shift gears, as Roche and Novartis were starting to offer industry postdoc positions to attract machine learning talent. "As long as you were skilled in computer science, coding, and machine learning, they would teach you the biology side," Jonathan explained. "I took that opportunity at Novartis."
There, Jonathan worked on generative AI to create synthetic patient data, including MRI, CT scans, and text records. This synthetic data allowed for collaboration and prototyping without risking patient privacy. He then continued as a researcher for another year and a half, focusing on multimodal computer vision projects related to neurodegenerative diseases like progressive multiple sclerosis and ophthalmological conditions like age-related macular degeneration.
"We were trying to develop machine learning models that could better understand retinal images than doctors or traditional models trained on general objects like cats, houses, or cars," he explained.
Moroccan conversations
Jonathan's journey to Cradle was somewhat unexpected. During a workshop in Morocco with academics and ML scientists from Google DeepMind, he found himself surrounded by brilliant minds solving complex problems in casual conversations. "I was sitting there, so impressed by the caliber of people there," he remembered. The discussions were enlightening, and someone mentioned Cradle. When he returned home, he contacted an old colleague who had joined the company, and his enthusiasm was infectious. "He just went off. He was like, 'It's amazing, this is such a great place. You should totally check it out.'" Jonathan laughed while mimicking his colleague's animated excitement.
A series of meetings with Cradle's CTO and CEO solidified his interest. Jonathan described his onsite visit, noting that he was impressed by the energy and talent in the room. "I came to visit and decided, okay, this is a team from which I can learn so much and where I can do really awesome stuff. And then it was a no-brainer for me to make the switch at that point," he said, clearly enthusiastic about joining Cradle's team.
Rethinking biology with ML
Coming from physics, Jonathan admits biology's messiness and uncertainty can be triggering. "Biology is so squishy and not exact in any way. It's something that definitely gets me once in a while, like, 'It can't be, this is our answer?!' There's so much uncertainty every step of the way, and we only have so few data points. It's something I have to get used to on a daily basis."
Despite this challenge, he sees the collaborative approach at Cradle between machine learning and lab as essential. "Instead of downloading trial data to work with like at Novartis, here I can speak with the people in the lab, be like 'Hey I want to test something, could you build this for me?' This interaction makes it much more interesting to build ideas, test these ideas, and get feedback from actual real-world experiments, which I really like."
Regarding the skepticism some biotech professionals have towards machine learning, Jonathan thinks it comes from overestimating human knowledge. "ML folks often try to introduce the knowledge of the world into models in certain ways, like 'We know it's this way, that's why we train it like this,'" he explained. "But then if you remove these assumptions, the models often perform better, just because our assumptions aren't complete, accurate, or only work for specific cases we've seen. This is very similar to what biologists struggle with - they only have sparse information on how biology works. All of us only have very sparse information on how biology works. That's why we're investigating it. So, it's really hard to question your assumptions and consider ML an augmentation, not a replacement. That's a really challenging thing to do."
He highlighted that Cradle is committed to collaborating with experts and respecting their insights. "If someone has strong opinions about something, we take those into consideration," Jonathan said. "It's not like we don't value years of experience or a PhD in biology. We're introducing pathways for their knowledge to inform our machine learning as well."
The cutting-edge of protein ML
In his multifaceted role as an ML Scientist at Cradle, Jonathan focuses on three key areas: staying abreast of scientific developments that could translate to the teams' work (even if not directly in the young field of generative protein engineering), exploring long-term research directions that may not pan out but push boundaries, and helping scope projects for new customers to match Cradle's current capabilities.
One of the recent scientific developments that particularly excites him is a new method for training models on lab data to predict protein sequences optimized for specific traits like melting temperature. "The way these generative models are trained now is very similar to how ChatGPT is trained on the entire corpus of the web. In the case of biology, you just show them examples from nature, hundreds of millions of sequences, and mask out elements for the model to predict," Jonathan explained. "This teaches it to suggest plausible protein sequences, but not necessarily ones better than what clients already have."
"With our new method, we now take lab measurements into account. For example, if one protein melted at 53 degrees Celsius and another at 47 degrees, the 53 one is clearly better if you're aiming for higher melting temperatures," he continued. "With this labeled data, the model doesn't just learn what a plausible sequence looks like, but what a better plausible sequence toward a goal would be. This is called supervised training, instead of the unsupervised or self-supervised training the models were doing before."
However, applying ML to protein optimization comes with significant challenges, particularly with data availability. "We have billions of unlabeled sequences, but labeled data - measurements from the lab - we often don't get that many," Jonathan said. "And getting new data usually takes 2-3 months, at most labs, because the lab has to grow the protein, express things, test it, run the assays. So, the turnaround times are slow, and the dataset sizes are tiny. Those are both very, very large challenges," he noted. However, Cradle has been working to cut this turnaround time down to just two weeks.
He likened it to stumbling around in the dark. "If you imagine all possible sequences as a landscape, we only have a few landmarks to navigate by, and we're trying to walk through it to find a hill," Jonathan explained. "It's very hard to know we're not making something up or getting lost on a little mound while the actual hill is super far away."
Another layer of complexity comes from biology's inherent variability and noise. "There are always measurement errors," he noted. "If you test on Monday, it might perform differently than on Wednesday, or if lab assistant A runs the assay versus lab assistant B. So it's really hard to compare different things, to know if we're comparing apples to apples."
Protecting IP
Given the substantial value of new pharmaceutical compounds—some of which can drive billions in revenue—Jonathan emphasized how critical data privacy and IP protection are in their field and how Cradle ensures client data stays siloed.
"A model is prepared with unsupervised learning on openly available data, data we've bought, or data we've gathered in our own labs, so it doesn't have any external IP," he explained. "But as soon as we start shifting a model's knowledge toward a client's project, that model is cut off from any other project. There's no way for information to leak between clients because, as our CEO rightly says, that's the easiest way to kill the company." “We have actually just published a blogpost about Data Privacy and Model Integrity”.
Going forward
Looking ahead, Jonathan is excited about the wide range of applications for Cradle's protein optimization technology. "It's fantastic to see companies doing amazing work with processes that use a fraction of the CO2 emissions—and sometimes even remove CO2 from the air," he said. "I definitely want to contribute to that." He also mentioned that new medications, treatments, and innovations in food and agriculture are equally intriguing. "There's so much happening. I think we're at the start of something incredible," he shared, suggesting that we might witness a "Biorevolution" that could give birth to thousands of new companies.
For those considering joining Cradle, Jonathan highlighted the unique learning opportunities. "No matter how good you are at what you're doing, you will learn new and exciting things here," he explained. "That's not something many places can offer. You'll also be working on really interesting projects, helping move society in the right direction at a pivotal point in time."
As our conversation wound down, we chatted about some of the lighter aspects of life at Cradle, like the frequent offsites (where a sauna or hot tub is always on the checklist) and Jonathan's go-to coffee order (a double-shot cappuccino in the morning and espressos throughout the day, with the occasional afternoon cappuccino for a bit of extra "spice").
Talking with Jonathan was both enjoyable and enlightening. The conversation offered a glimpse into the world of a multidisciplinary scientist who applies his diverse skills to push the boundaries of what's possible. His journey from audio engineering to protein optimization illustrates how unexpected paths can open when you embrace curiosity, challenge yourself, and remain open to new possibilities. It's a reminder that innovation often emerges at the crossroads of different disciplines—and that there's always room for those willing to explore.
Before the interview series with Cradlers kicked off, a colleague asked me an interesting question: "Do you want to interview a biologist or an ML scientist?" My response was short: 'It doesn't really matter to me,' and had two reasons behind it. Firstly, I had never conducted interviews before, so whether the conversation was about cutting-edge biology or advanced machine learning, I was bound to learn something new. Secondly, the biotech industry has entered a transformative era where the lines between life scientists and tech experts are blurring. Biologists are embracing technology and exploring computational methods, while engineers are delving into the complexities of life sciences. This merging of fields means that regardless of who I interviewed, the conversation would explore a fascinating intersection of knowledge and innovation.
Following that profound monologue in my mind, a common occurrence after discussions or altercations, where one creates the most fitting and extraordinary argument—a masterpiece worthy of a Pulitzer Prize in Explanatory Reporting—merely two weeks later, I found myself in a Google Meet session with Jonathan, Cradle's resident ML scientist. Or, as Google labeled him, "CH-ZRH-BLE-4-Rosalind Franklin," as each meeting room at Cradle is named after pioneering women in science and engineering.
Harmonizing disciplines
Jonathan is the ideal example of the convergence between technology and biology. His background is an eclectic mix of music, technology, and now biology—a physicist, music producer, ML scientist, and more.
When I asked him how he ended up with such an intriguing combination of skills, Jonathan revealed that his early years were all about music. "Music came first," he said, recalling how he began playing the violin at five years old. His parents were professional classical musicians, and his sister is now a professional singer. Despite his musical upbringing, Jonathan was drawn to STEM subjects, describing himself as "the oddball" fascinated by math, science, and technology.
Although Jonathan decided to study physics as a teenager, he quickly found himself questioning that choice once he entered university. "I found myself at university, enrolled in physics, and I was like, what the hell am I doing here?" he said with a smile. To find a new direction, he interned at SWR, a German government radio station with multiple orchestras and a studio for experimental contemporary live electronic music. "Lots of noise, screeching, weird sounds, and things like that," he described. This experience uncovered something unexpected: the resident sound directors knew more about physics than he did. "I was a physicist, and they were musicians, but they knew more about physics than I did," Jonathan laughed.
This realization led him to also study music informatics at a conservatory, blending technology and music. After graduation, he pursued music production, but the routine eventually wore him down. "It's the same thing every weekend: you go somewhere, you sit backstage, you wait for the sound check, you do the sound check, you play the concert, you go to the hotel, you go to the next venue, and so on," he said, describing the monotonous cycle that made him rethink his path. The repetitive nature of the music industry pushed him back to academia, where he earned a PhD in computer science.
Pioneering ML for audio
During his PhD, Jonathan initially focused on multi-microphone setups for large venues and events. At some point, he realized that machine learning could solve many of the problems he was tackling. "The tasks I was painstakingly attempting to figure out were far more effectively solved by machine learning," he shared. This realization prompted Jonathan to shift focus, allowing him to secure additional funding, including a grant to form a small team and become a pioneer of ML research at his university.
"We established the Institute for Applied Artificial Intelligence during that period, and it was fantastic," he explained. "It was mostly graduate students and professors, and we didn't do any teaching—just pure research. I was essentially the audio guy. We had people working on computer vision, natural language processing, and analyzing Japanese comics from an ML perspective—quite a range of disciplines, including some in medicine. It was an incredibly interesting time."
From audio to pharma
After completing his research at the institute, Jonathan realized his ML skills could have a broader societal impact beyond audio applications. He considered focusing on either life sciences or environmental sciences, but personal and family reasons steered him toward medicine. "Unfortunately, I've been in very close contact with a lot of medicine, so it really got me hooked," he said, adding with a grin, "The research, not the meds."
It was a great time to shift gears, as Roche and Novartis were starting to offer industry postdoc positions to attract machine learning talent. "As long as you were skilled in computer science, coding, and machine learning, they would teach you the biology side," Jonathan explained. "I took that opportunity at Novartis."
There, Jonathan worked on generative AI to create synthetic patient data, including MRI, CT scans, and text records. This synthetic data allowed for collaboration and prototyping without risking patient privacy. He then continued as a researcher for another year and a half, focusing on multimodal computer vision projects related to neurodegenerative diseases like progressive multiple sclerosis and ophthalmological conditions like age-related macular degeneration.
"We were trying to develop machine learning models that could better understand retinal images than doctors or traditional models trained on general objects like cats, houses, or cars," he explained.
Moroccan conversations
Jonathan's journey to Cradle was somewhat unexpected. During a workshop in Morocco with academics and ML scientists from Google DeepMind, he found himself surrounded by brilliant minds solving complex problems in casual conversations. "I was sitting there, so impressed by the caliber of people there," he remembered. The discussions were enlightening, and someone mentioned Cradle. When he returned home, he contacted an old colleague who had joined the company, and his enthusiasm was infectious. "He just went off. He was like, 'It's amazing, this is such a great place. You should totally check it out.'" Jonathan laughed while mimicking his colleague's animated excitement.
A series of meetings with Cradle's CTO and CEO solidified his interest. Jonathan described his onsite visit, noting that he was impressed by the energy and talent in the room. "I came to visit and decided, okay, this is a team from which I can learn so much and where I can do really awesome stuff. And then it was a no-brainer for me to make the switch at that point," he said, clearly enthusiastic about joining Cradle's team.
Rethinking biology with ML
Coming from physics, Jonathan admits biology's messiness and uncertainty can be triggering. "Biology is so squishy and not exact in any way. It's something that definitely gets me once in a while, like, 'It can't be, this is our answer?!' There's so much uncertainty every step of the way, and we only have so few data points. It's something I have to get used to on a daily basis."
Despite this challenge, he sees the collaborative approach at Cradle between machine learning and lab as essential. "Instead of downloading trial data to work with like at Novartis, here I can speak with the people in the lab, be like 'Hey I want to test something, could you build this for me?' This interaction makes it much more interesting to build ideas, test these ideas, and get feedback from actual real-world experiments, which I really like."
Regarding the skepticism some biotech professionals have towards machine learning, Jonathan thinks it comes from overestimating human knowledge. "ML folks often try to introduce the knowledge of the world into models in certain ways, like 'We know it's this way, that's why we train it like this,'" he explained. "But then if you remove these assumptions, the models often perform better, just because our assumptions aren't complete, accurate, or only work for specific cases we've seen. This is very similar to what biologists struggle with - they only have sparse information on how biology works. All of us only have very sparse information on how biology works. That's why we're investigating it. So, it's really hard to question your assumptions and consider ML an augmentation, not a replacement. That's a really challenging thing to do."
He highlighted that Cradle is committed to collaborating with experts and respecting their insights. "If someone has strong opinions about something, we take those into consideration," Jonathan said. "It's not like we don't value years of experience or a PhD in biology. We're introducing pathways for their knowledge to inform our machine learning as well."
The cutting-edge of protein ML
In his multifaceted role as an ML Scientist at Cradle, Jonathan focuses on three key areas: staying abreast of scientific developments that could translate to the teams' work (even if not directly in the young field of generative protein engineering), exploring long-term research directions that may not pan out but push boundaries, and helping scope projects for new customers to match Cradle's current capabilities.
One of the recent scientific developments that particularly excites him is a new method for training models on lab data to predict protein sequences optimized for specific traits like melting temperature. "The way these generative models are trained now is very similar to how ChatGPT is trained on the entire corpus of the web. In the case of biology, you just show them examples from nature, hundreds of millions of sequences, and mask out elements for the model to predict," Jonathan explained. "This teaches it to suggest plausible protein sequences, but not necessarily ones better than what clients already have."
"With our new method, we now take lab measurements into account. For example, if one protein melted at 53 degrees Celsius and another at 47 degrees, the 53 one is clearly better if you're aiming for higher melting temperatures," he continued. "With this labeled data, the model doesn't just learn what a plausible sequence looks like, but what a better plausible sequence toward a goal would be. This is called supervised training, instead of the unsupervised or self-supervised training the models were doing before."
However, applying ML to protein optimization comes with significant challenges, particularly with data availability. "We have billions of unlabeled sequences, but labeled data - measurements from the lab - we often don't get that many," Jonathan said. "And getting new data usually takes 2-3 months, at most labs, because the lab has to grow the protein, express things, test it, run the assays. So, the turnaround times are slow, and the dataset sizes are tiny. Those are both very, very large challenges," he noted. However, Cradle has been working to cut this turnaround time down to just two weeks.
He likened it to stumbling around in the dark. "If you imagine all possible sequences as a landscape, we only have a few landmarks to navigate by, and we're trying to walk through it to find a hill," Jonathan explained. "It's very hard to know we're not making something up or getting lost on a little mound while the actual hill is super far away."
Another layer of complexity comes from biology's inherent variability and noise. "There are always measurement errors," he noted. "If you test on Monday, it might perform differently than on Wednesday, or if lab assistant A runs the assay versus lab assistant B. So it's really hard to compare different things, to know if we're comparing apples to apples."
Protecting IP
Given the substantial value of new pharmaceutical compounds—some of which can drive billions in revenue—Jonathan emphasized how critical data privacy and IP protection are in their field and how Cradle ensures client data stays siloed.
"A model is prepared with unsupervised learning on openly available data, data we've bought, or data we've gathered in our own labs, so it doesn't have any external IP," he explained. "But as soon as we start shifting a model's knowledge toward a client's project, that model is cut off from any other project. There's no way for information to leak between clients because, as our CEO rightly says, that's the easiest way to kill the company." “We have actually just published a blogpost about Data Privacy and Model Integrity”.
Going forward
Looking ahead, Jonathan is excited about the wide range of applications for Cradle's protein optimization technology. "It's fantastic to see companies doing amazing work with processes that use a fraction of the CO2 emissions—and sometimes even remove CO2 from the air," he said. "I definitely want to contribute to that." He also mentioned that new medications, treatments, and innovations in food and agriculture are equally intriguing. "There's so much happening. I think we're at the start of something incredible," he shared, suggesting that we might witness a "Biorevolution" that could give birth to thousands of new companies.
For those considering joining Cradle, Jonathan highlighted the unique learning opportunities. "No matter how good you are at what you're doing, you will learn new and exciting things here," he explained. "That's not something many places can offer. You'll also be working on really interesting projects, helping move society in the right direction at a pivotal point in time."
As our conversation wound down, we chatted about some of the lighter aspects of life at Cradle, like the frequent offsites (where a sauna or hot tub is always on the checklist) and Jonathan's go-to coffee order (a double-shot cappuccino in the morning and espressos throughout the day, with the occasional afternoon cappuccino for a bit of extra "spice").
Talking with Jonathan was both enjoyable and enlightening. The conversation offered a glimpse into the world of a multidisciplinary scientist who applies his diverse skills to push the boundaries of what's possible. His journey from audio engineering to protein optimization illustrates how unexpected paths can open when you embrace curiosity, challenge yourself, and remain open to new possibilities. It's a reminder that innovation often emerges at the crossroads of different disciplines—and that there's always room for those willing to explore.
Subscribe to updates
Get new posts and other Cradle updates directly to your inbox. No spam :)
Recent posts
Built with ❤️ in Amsterdam & Zurich
Built with ❤️ in Amsterdam & Zurich